This study aims to use problem solving techniques in solving problems at different stages step by step. The theoretical and experimental semiotic model was developed by using the semiotic model method, which can be established with the relation of priority-recency, and an analysis of the problem solving skills of the students was made. Given that the procedural knowledge questions are used as the type of question to be applied in the development of the semiotic model, the model to be developed is called the semiotic procedural knowledge model. The experimental model will be established with the data collected from 34 science teacher candidates by means of using the qualitative method. Comparing the theoretical and experimental model, analyses will be made to compare the students' knowledge levels and success levels in the steps used correctly or incompletely in problem solutions. These analyses reflect students' ability to establish a relationship between scientific knowledge and technology.
The first work in science education is the teaching of science. Naturally, science teachers place the building stones of science for individuals. Science can be taken as a process of knowing and / or understanding for individuals in educational processes. However, it can be said that individuals or institutions involved in educational processes have an explicit or implicit righteous expectation from teaching science, as well as teaching the application of science. A strong bridge can be established between science and its applications through the use of questions and problems.
Scientific knowledge can be expressed with different types of knowledge. One of the types of knowledge in which scientific knowledge can be expressed is the procedural knowledge. Researches show that science teachers and teacher candidates experience problems in the areas of conceptual knowledge and procedural knowledge. One of the sources of these problems is the inability to meet the educational needs of teachers at universities adequately (Verdugo and Solaz, 2019), while the other is the initial level of knowledge, that is pre-university stages (Tapia et al., 2019). Clearly there is overlap between the teaching of science and other areas of knowledge. We must constantly develop new methods to teach and differentiate between science education and teaching science in response to the changing needs of our students (Callahan and Dopico, 2016).
Procedural knowledge is one of the knowledge that is needed to understand concepts and develop problem solutions. Procedural knowledge is the knowledge of working procedures, steps and how to solve problems according to these steps (Wuryaningrum et al., 2020). Procedural knowledge can be defined as knowledge that includes sequential action steps and the application of automated techniques to solve problems (Aydı and Özgeldi, 2019). Apart from the situational and conceptual knowledge, procedural knowledge (including solution strategies) is of particular interest, but since it is much more difficult to measure, refined procedures should be developed for this type of knowledge, including a suitable benchmark to measure and evaluate procedural knowledge (Richter-Beuschel et al., 2018).
Like other topics in science, students’ success in solving the equation depends on the learners’ grasp of certain types of knowledge in equation solving (Rittle-Johnson et al., 2001). These two types of knowledge in equation solving may not be clearly separated from each other. The interplay between procedural and conceptual knowledge occurs in the procedural step when the learner selects an operation to change the state of the equation and yet at the same time to maintain the equality of the equation (Ngu and Phan, 2016). Experts in certain disciplines often choose appropriate procedures to produce an efficient or elegant solution to a mathematical task. This flexible procedural knowledge distinguishes novice and expert procedural performances (Maciejewski and Star, 2016).
With PSTs, the mathematical thinking of students can be affected in a positive manner (Singh et al., 2018). Various evidences have been presented on the matter that thinking is an effective tool for students to improve their knowledge structures. By means of thinking, a student tries to explain and create a subject during the learning process (Sarwar and Trumpower, 2015). However, while solving tasks that are directed as conceptual and procedural comprising of length, area and mass measurement, the students may experience conceptual mistakes and faults. Such basic conceptual mistakes indicate that the students experienced difficulty in understanding the relations, along with the procedures ad formulas used for measurement (Tan and Aksu, 2016). When students are asked to map certain knowledge used in the teaching processes by means of using the mapping strategies, the teachers can be provided with substantial support in terms of understanding the units (Giamellaro et al., 2017). Within the scope of solving complicated problems, causal mapping can be used in order for strategic decisions actions to be supported (Öllinger et al., 2015). The teachers can successfully guide their students through open questioning processes by means of addressing the conceptual, epistemic, social and/or procedural areas (van Uum et al., 2016).
Problem solving techniques (PST)
Problem solving techniques can be created from specific phase and steps. Students can solve these problems by applying these phases step by step. In the problem-solving phase, it can activate students' previous knowledge, become aware of knowledge gaps, and discover deep features of the target content, which can prepare them to better handle the next instruction (Loibl et al., 2020).
PSTs can be developed in line with various purposes. In addition to differing from each other, they also differ in terms of problem detection approaches, solving techniques and interpretation of results within various disciplines. To give an example, developed by means of the effects of four-stage Polya problem solving technique (Yong et al., 2018; Okafor, 2019), empathy-based problem solving technique (Lewis et al., 2019), problem solving technique oriented at metacognition measurement (Haeruddin and Supahar, 2020), problem solving techniques of representative technologies (McCollum et al., 2016) or problem solving of causal mapping (Öllinger et al., 2015), PSTs differ from each other in terms of both improvement purposes and criteria. Additionally, brain-storming based creative problem solving techniqes comprise five steps: “CPS fiveâ€step model, comprising Factâ€Finding, Problemâ€Finding, Ideaâ€Finding, Solutionâ€Finding and Acceptanceâ€Finding” (Sousa et al., 2014a). On the other hand, small group problem solving technique has also been developed by means of adopting the four stages of creative problem solving techniques into the group studies (Sousa et al., 2014b).
When PST is developed by means of dividing the problem into parts and solving it as step by step, priority-recency relations can be established between the steps of this tecnique. This technique generally comprises inputs-outputs for (G-A), free body diagram (FBD), formula, definition and process steps (Yılmaz, 2016a, 2016b, 2014, 2012). This step can be used more effectively in the problem solving of courses like biology or those of social content, since the definitions are not substantially used within the numerical problem solving processes in courses like physics, mathematics, etc. By means of utilizing the priority-recency relations of problem solving steps, the semiotic model can be established, as well.
Semiotic procedural knowledge model (SPKM)
By increasing the visualization of knowledge in science as both process and product, the discovery of the roles of these methods has accelerated. It is a visual method in drawing. Researchers position this method as a potential key for reasoning and learning in teaching environments (Tytler et al., 2020). In drawing studies, markings can be made for different purposes. It is used to: record dissections (Panagiotopoulos et al., 2016), translate text into an image (Leopold and Leutner, 2012; Brooks, 2009), interpret processes (Hubber et al., 2010), and enact speculative accounts of unfamiliar or abstract phenomena (Prain and Tytler, 2012; Ainsworth et al., 2011).
Semiotic model is based upon the empirical findings of Benjamin Libet in the neurophysiology of sensory awareness (Cantor, 2014a). Semiotic model is used by a number of different disciplines in line with the objectives of organizing the knowledge, convenient interpretation of the same and/ or rendering the knowledge more accessible. Just like a semiotic model can be developed for detecting the optimal character required for the sculpture placement area in order for an artist to ensure that the conceptions are transmitted to the audience (Erman and Boran, 2015), the same can be done for repsenting the mental processes (Cantor, 2014b), along with modelling, representing and interpreting various meical concepts (Kwiatkowska and Kielan, 2013), orgnaizing the problem solving (Roux and Kloot, 2019; Yılmaz, 2016a) or organizing the knowledge (Reid et al., 2019). There is a critical concept of semiotic modeling that directly addresses the core of the philosophy of education in the light of semiotics and affords valuable implications for the nascent interdisciplinary field of inquiry of edusemiotics or educational semiotics (Yu, 2017) Models provide a structure for organizing knowledge and facilitating learning (Reid et al., 2019). Problem solving is modeled in order to overcome the problems faced by students in problem solutions (Roux and Kloot, 2019).
The semiotic model of procedural knowledge, which has a crucial role in scientific knowledge, priority-precency relations of PST steps, pictorial diagrams (Özenli, 1999) and semantic networks (Özenli, 1999) can be used to constitute SPKM (Yılmaz, 2016a, b). Since SPKM will be prepared considering the priority-recency of PST steps, it can be more advantageous in teaching processes compared to other models. For problem solving of this research, a five-step semiotic model can be established, which are I-O, FBD, formula, process steps and the result of problem solving of the PST based on dividing the problem into separate parts, and solving step by step (Yılmaz, 2016a, b). The G-A step is used in the beginning of priority-recency relations at the I-O step for establishing the semiotic model, since it is possible to start with I-O step where the inputs and outputs are determined in the question at first for solving the problem. The next step within the priority-recency relation for developing the semiotic model is the FBD and/or formula, where the formulas are used in the process step for solving the problem. The problems to ensure the establishing of such semiotic model based on problem solving are limited to certain type of knowledge; this semiotic model becomes the model of that type of knowledge, as well. Rendering the problem solving-based semiotic model to be a procedural model can be ensured with three steps of PST. The first one will be created with the inputs and outputs of the problem. The hidden inputs of the problem should be able to be identified with open procedures within the I-O step. The second one is the free body diagram as one of the steps to provide convenience in process steps within the scope of identifying the required relation or formulas on the process step of the problem. The relation and/or formulas should be able to be identified through open procedures on the FBD step, as well. The third one is that the formulas should be able to be identified through open procedures in the formula step where the formulas that lead to the result can be found out with the inputs at the process step of the problem. In this way, a theoretical SPKM can be established based on problem solving, when these three steps are created with the processes that can be identified with open procedures. Since the elements of the measurement tools used in the research are limited to procedural knowledge problems, this model is named as SPKM (Figure 1).
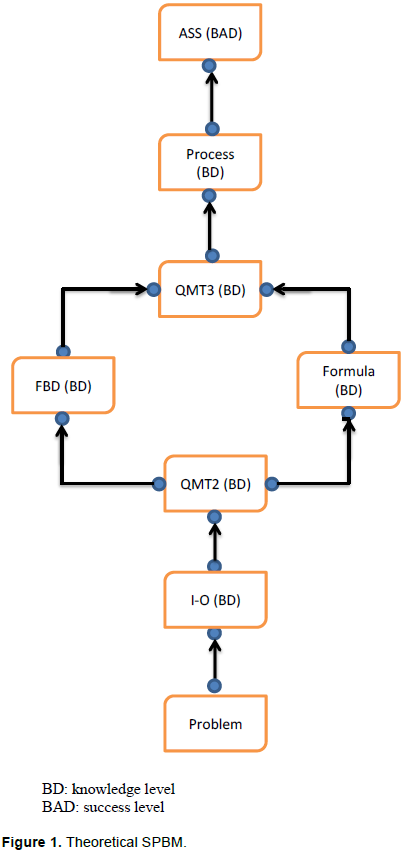
Knowledge-centered measurement
The measurement tools used in education can be grouped into two main categories, either individually or knowledge-centered, based on what is to be measured or the superior purpose of measurement (Yılmaz, 2020). With the individual-centered measuring tools (classical measuring tools), the learning speed of individuals (students) in the educational process can be determined. With the individual-centered measurement, more contribution can be made into the development of appropriate teaching environments and methods that will increase the learning speed of individuals. With the individual-centered measurement, a controlled contribution can be made to better the education of individuals. Knowledge centered measurements can be made with probability measurement tools. Probabilistic measurement tools can be evaluated with knowledge theories or VDOIHI method, where probabilistic evaluation can be made (Yılmaz, 2020). Probabilistic evaluation gives how much of the knowledge an individual needs to accomplish. In this science teaching, it allows us to analyze how students can relate to scientific knowledge and technology. In professional life, it is important as much as the learning speed or capacities of individuals and how much knowledge they need to apply. It is important for the development of humanity to be expected from science education that individuals or institutions involved in education and training processes can also be able to practice science. Individuals can contribute to both themselves and the development of humanity in similar ways as much as they can transform their knowledge into practice.
Objective
Equation approach based on two-step problem solving processes is a dominant strategy adopted by teacher candidates since it brings along low cognitive load (Ngu, 2019). Notwithstanding that this approach is a strategy used predominantly by teacher candidates, it cannot be said that sufficient studies have been carried out showing the effect of teacher candidates on their knowledge and success levels. It has been suggested that students have a low level of knowledge about certain subjects before university education, and in this aspect they become clearer when subjected to procedural and / or comprehension research. In addition, after completing the subject lesson in which they have a low level of knowledge, the level of knowledge increased significantly, but in some of the skills and competencies of stdents that need to be solved, basic conceptual errors and significant uncertainties have still been found (Tapia et al., 2019).
In this research, it is aimed for individuals to establish a strong relationship between science and technology, and the difficulties and deficiencies encountered in establishing this relationship can be determined by SPKM based on PST and contribute to the development of education with a new modeling. Theoretical and experimental semiotic model will be developed by using the semiotic model method, which can be established with the priority-recency relation of the steps of the PST; it is based on solving a problem step by step at certain stages, and the analysis of the problem solutions of first-year science students will be made, as well.
The data of the study were collected from 34 science teachers’ first year students. PST, used in problem solving before data are collected, has been taught and applied to first grade students. After the teaching of the PST, the related data were collected with three measurement tools that were validated in the teaching of the subjects; they are related to the questions of the measurement tool, without creating the ideal teaching environments specific to the research, after completing the subject processing in the natural teaching environment. The items of the three measurement tools were formed by open ended questions. The answer key for each measurement tool has been prepared. Each item of the answer keys prepared for the measurement tools was allocated into the PST steps as per the VDOIHI method. PST steps have been digitized through the VDOIGI method. The score “1” is used for digitization through this method by means of dividing the steps into the smallest significant parts. Comparing the digitized answer keys and the data collected from the students, the data digitization has been performed. The scores “-1, 0, 1” are used for the digitization of the data. Where the smallest significant part found in the data matches with the answer key, the score to be given is “1”. The score to be given is “-1”, where they do not match. For the smallest significant parts that are in the answer key, yet not found in the data collected, the score to be given is “0”. Probability assessments will be carried out with the numerical values of these scores. This is a knowledge-centered assessment since to-be (the smallest significant parts of the answer key) and as-is (the smallest significant parts of the data) are subjected to assessment through probability processes in terms of their smallest significant parts through this method. Since the critical questions regarding what they need in procedural knowledge are required to be evaluated so that students can be informed about scientific practices (Kuhn, 2016), the first measurement tool created from questions related to the applications of students' scientific knowledge in technology and data were collected after the other two measurement tools were applied. The data of the research were collected from work and kinetic energy issues. In the analysis of the data obtained from three measurement tools, VDOIHI method and the package program of this method are used, where objective logic analysis of qualitative data can be made. From the data to be collected with the PST, the knowledge and achievement levels of the students can be analyzed with the VDOIHI method. The analysis of the qualitative data of the PST reflects students' ability to establish a relationship between scientific knowledge and technology.
Measurement tools
Three measuring tools were used in the research. The first (basic measurement tool) (QMT1) is the measurement tool in which students' level of knowledge and success can be determined in the steps of the PST. This measurement tool is the one that will use the steps of the PST in the establishment of the experimental semiotic model. The questions of the measurement tool consist of those related to the applications of students' procedural knowledge on technology in work and energy. There are 10 open-ended questions in this measurement tool that are validated and can be solved by the application of the PST. The steps of the PST that can be applied for each question of the basic measurement tool can be seen in Table 1.
The second measurement tool (QMT2) consists of 15 open-ended questions asking for the equations to be used in solving the problems of QMT1. Since this measurement tool is formed from the equations that should be given by the students in the formula and / or FBD digit of the basic measurement tool, the data of this measurement tool can be used in the analysis of the data of the formula, FBD and process steps of the basic measurement tool.
The third measurement tool (QMT3) focuses on measuring the mathematical logic structures of the operations in the process step of QMT1. In the questions of this measurement tool, mathematical propositions related to work and energy are given first. Then, with these propositions, questions related to mathematics and logic were asked. This measurement tool consists of 10 open-ended questions that have been validated. With this measurement tool, students' level of knowledge and success levels can be analyzed in the process level of QMT1.
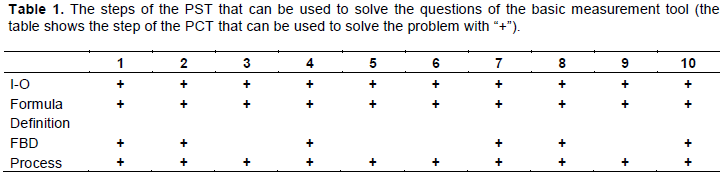
Assessment method
VDOIHI method will be used in the analysis of the data obtained by measuring tools. With this method, objective logic analysis of the data collected with qualitative measurement tools can be made. VDOIHI method can be used for qualitative data by digitizing the data. Symmetrical possibilities are calculated through the digitization of the qualitative data obtained by the measurement tool via the VDOIHI method. Then, by digitizing the data of the answer key, probability distribution numbers are calculated. Later on, symmetrical probabilities can be analyzed by obtaining findings in the section on probability distribution numbers. Where the findings belong to the problem solving steps, it gives the level of knowledge, and if it belongs to the result of the problem, it gives the success levels. VDOIHI combined step method will be used in the analysis of the data of this research (Yılmaz and Yalçın, 2011; Yılmaz, 2011). In the combined stage method of VDOIHI, if there is more than one stage in one section, these stages are converted into a single stage. This conversion process can be done with mathematical processes. For this, all scores of the same type in the stages of a section are collected. Since two scores are used in the digitization of the data in the VDOIHI combined step method, evaluation is made with two-based probability processes (Yılmaz and Yalçın, 2011; Yılmaz, 2011, 2017). Combination equation is used in these processes.
In order to analyze qualitative measurement tools with the VDOIHI method, measurement tools must have answer keys. VDOIHI method gives a score to the smallest meaningful parts in the answer key. If this method is to be applied to the PST, each step of the PST is defined as a section in this method. Each section can consist of multiple stages. From the sum of the meaningful smallest piece scores in the answer key, the score that can be taken at one stage is determined. By comparing the data obtained as a result of the application with the answer key, 0 or ∓ 1 scores are given to the smallest meaningful parts of the application data. The same type of scores of all stages in one part of the application data are summed and grouped and their scores are obtained, as well. These scores are taken as the number of symmetrical states of probability distributions. The sum of the scores in one part of the answer key is taken as the number of cases and the number of events. In the combination equation, symmetrical probabilities are calculated from the number of symmetrical states from 0 to the number of symmetrical states obtained from application data. Then the calculated symmetrical probabilities are summed up. Knowledge or success levels are calculated from the sum of the total symmetric probability divided by the number of probability distributions (Yılmaz and Yalçın, 2011; Yılmaz, 2011, 2017). If these processes are performed for the steps of the PST, the score obtained gives the level of knowledge. Where they are performed for the result of the problem, the score obtained gives the level of success.
Experimental SPKM
In the establishment of the experimental model, the theoretical model, of which PST was established in priority-recency order, will be used. If the score (knowledge level) in the problem solving step (section) is between 0 and 0.1 (0≤ knowledge level≤0.1), it will be regarded as an unused step and the semiotic relationship will not be shown because that step is used on a quite low level by the students. If the score obtained in the problem solving step is between 0.1 and 0.7 (0.1 <knowledge level≤0.7), it will be accepted that the step is used at the intermediate level by students and the semiotic relation will be shown with a dashed line {⇢}. If the score obtained in the problem-solving step is between 0.7 and 1.0 (0.7 <knowledge level≤1.0), it will be accepted that the step is used well by the students and the semiotic relationship will be shown with a straight line {⟶}. Thus, the representation of the semiotic model includes knowledge about the students' level of knowledge and achievement levels.
The data obtained from QMT1, which consists of 10 open-ended procedural knowledge questions directed to 34 science teacher candidates in order to determine the level of knowledge and success in the students' PST steps, are given in the first five lines of Table 2. These findings show students' level of knowledge on work and energy. The data obtained with QMT2 through 15 open-ended questions asking for the equations to be used in solving the problems of QMT1, is obtained in the sixth row of Table 2. These findings show the level of knowledge of the students regarding the procedures of the formulas on work and energy problems. The data obtained with QMT3 in the process step of QMT1 created with 10 open-ended questions in order to determine the mathematical logic structures of processs is given in the seventh line of Table 2. These findings show students’ mathematical logic knowledge level in work and energy problems. From the comparison of the result obtained in the process step with the result of the answer key, the finding obtained by the combined VDOIHI method is given in the eighth line of Table 2. This finding shows the success level of students in work and energy problems.
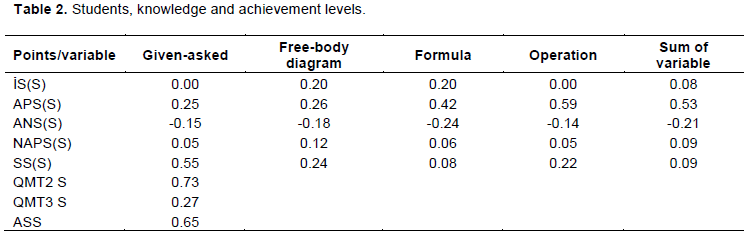
The unrelated scores (US) obtained with QMT1 are given in the first row of Table 2; they are low for all variables (0.00≤US≤0.20). The students have negative scores in all significant smallest parts of a stage (unrelated). This indicates low levels of knowledge. The fact that the score of US in the I-O level is 0.00 indicates that the students do not establish irrelevant relationships in breaking down the problem. The most critical stage in the solution of a problem is the initial step; if unrelated relations are established in the initial step, the problem cannot be solved or the solution is rendered difficult by performing extra processes at the other steps of the PST. Although the students' formula knowledge level in the sixth row of Table 2, with work and energy obtained with QMT2 is as high as 0.73, students' 0.20 unrelated knowledge in the formula level given in the first row of Table 2 indicates that they have problems in associating formulas with problem. Similarly, the fact that QMT1 has an unrelated level of knowledge of 0.20 in the FBD level suggests that the positive scores (APS) in the formula level of the students decreases to a lower level of knowledge “0.42” in QMT2. In other words, they cannot associate the formulas they know with the problem in parallel with their knowledge due to the unrelated level of knowledge of 0.20.
The positive scores (APS) obtained in QMT1 and given in the second row of Table 2 show the positive knowledge levels of students on work and energy problems. It can be seen that students' positive knowledge level is on a medium level (0.25≤APS≤0.59). The fact that students' positive knowledge level in the process step of QMT1 is higher than all other levels of QMT1 indicates that the students are focused on the solution. It can also be seen that QMT2 does not reflect the knowledge levels of 0.73 to the positive knowledge levels of QMT1 in FBD and formula steps. This can be explained by the fact that students are focused on the solution. The students who focus on the solution and have a knowledge level of 0.73 from QMT2 have a low level of mathematical logic of knowledge, - 0.27 in QMT3; this shows the logic of these formulas. This may be the reason for not exceeding positive knowledge score of 0.59. Correct (NAPS) knowledge levels found in negative stages can be added to positive knowledge levels, as well. NAPS knowledge levels (0.05≤NAPS≤0.12) in all steps of QMT1 are significantly low.
Negative scores (ANS) obtained with QMT1 and given in the third row of Table 2 show the students' negative knowledge levels about work and energy. It can be seen that students' negative knowledge levels are low (0.14≤APS≤0.24). Low levels of negative knowledge indicate that less time can be devoted to misconceptions of concept-formula-knowledge in educational processes.
The success levels of the students are given in the last line of Table 2. The ASS score sows the students’ level of success in work and energy. The students' success level is on a medium level, as it has a score of 0.65. It is noteworthy that the success levels are higher than the positive knowledge level (APS) in the process level of QMT1. Achieving more success levels with less processing accuracy indicates that students carry out certain operations with their minds without writing. The fact that students have low levels of knowledge in the I-O, FBD and formula steps also shows that they focus on the result and therefore execute certain operations with reason without writing. This indicates that their students have more potential than measured at both their knowledge and achievement levels.
The level of knowledge and achievement obtained in this research is identified as moderate both in the study conducted with university students in similar teaching processes; teacher candidates having difficulties in the items that should bring together certain knowledge (Aydın and Özgeldi, 2019) and that students having difficulties in understanding basic relationships and formulas (Tan Sisman and Aksu, 2016) show that university students have problems in their previous education experience (Tapia et al., 2019; Tapia et al., 2019).
Models are developed for problem solutions so that problem solutions can be better understood by students and constructive contributions can be made to those who carry out teaching processes (Roux and Kloot, 2019; Salloum and BouJaoude, 2019; Öllinger et al., 2015). In addition, evidence of the positive contribution of these models has been obtained (Roux and Kloot, 2019; Salloum and BouJaoude, 2019). However, it has been conferred in the evidence that modeling created with causal mapping in the solution of complex problems is not effective in students' problem solving (Öllinger et al., 2015). This evidence shows us that care should be taken in developing models. In modeling the problem solving steps, it may be a good approach to develop a model with priority-recency relations that enable the establishment of the semiotic model. The relations shown in the theoretical SPKM, which was established with the priority-recency relations of the problem solving steps in the introduction section, can be established through experimental SPKM by drawing on the same model again by using experimental findings. Experimental data show the relations that students actually use and the priority-recency orders they use in these relations. Experimental SPKM can be established with students' positive knowledge levels. In Table 2, where the findings obtained from the data collected from 34 science teacher candidates with qualitative method are given, the experimental SPKM developed using the lines where the positive knowledge levels of the students are shown in the method section (Figure 2). They used the relations given in the theoretical model at an intermediate level, since all the positive knowledge levels of the students in the second row of Table 2 remained between 0.1 and 0.7. Experimental data show that there is a new relation that is not directly shown in the theoretical model. The fact that the knowledge level determined by the students with QMT2 is greater than 0.7 and that the highest positive level of knowledge in the steps of the PST is obtained in the process level shows that the students usually make a problem solution by establishing a relation between these steps. The use of other levels at a medium level may be the reason for the success levels to remain at a medium level (0.65). It can be said that the SPKM given in the above figure provides a holistic meaning to the students both in where they experience problems in problem solving and in what level they can use the relations between the steps. This can both help a student to better realize his or her academic level, and other elements of education to see the student’s academic status more holistically.
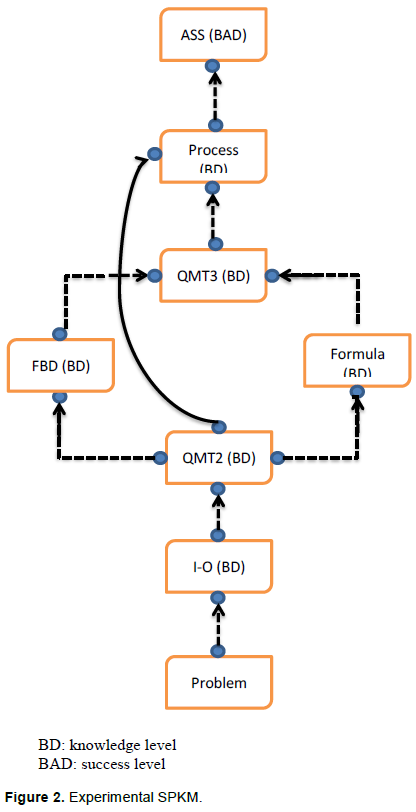
With the data collected through this study, it can be said that students have a medium level of positive knowledge at all levels of the PST and can establish a stronger relation between the formula knowledge level determined by QMT2 and the process steps. In other studies with first grade science students, positive knowledge levels were found to be low in the steps of the PST (Yılmaz, 2016a, b). Also in these studies, it was found that the relations between the knowledge level of the formula and the process step determined by the QMT2 in the semiotic knowledge models of the students were higher than the other relations. Based on these results, it can be said that the students studying in the first grade of science teaching focus more on the solution than understanding the problem and this focal point negatively affects their success levels. The fact that students focus both on the solution and their level of success shows that they have the potential to establish a medium level relationship between their scientific knowledge and technology.
The positive level of knowledge and achievement of the students shows that the level of problem solving should be developed. Since collaboration has positive effects on students’ problem-solving approaches (Birisci, 2017; Suarsana et al., 2019), problem-solving activities that they can collaborate can positively affect students' level of knowledge and sucess. Since problem solving skills (Latifah et al., 2019) and metacognitive awareness of procedural knowledge can be increased with the problem-based learning model (Kuvac and Koc, 2019), problem-based learning model can be used to develop positive knowledge levels. Given that visualization technique improves problem solving success levels (Osman et al., 2018), when visualization and modeling of problems affect problem solving successes (Roux and Kloot, 2019; Öllinger et al., 2015), establishing semiotic models of problem solutions can positively affect students' success levels.
The positive knowledge level of the students is the two lowest PST levels, I-O (0.25) and FBD (0.26) levels. In order to increase the knowledge level of students in the I-O step, they can spend more time to understand the question by breaking it into separate parts, provided that time efficiency is enabled in the other steps. There may be more than one reason for the low level of knowledge in the FBD step: not focusing on the solution and devoting enough care and time to this step; not being able to establish relationships between variables, having problems in shape drawings, etc. Collaborative learning strategies can also be used to alleviate the difficulties experienced in the FBD step, since collaborative learning strategies provide positive results in alleviating the difficulties experienced by students (Adeyemi and Cishe, 2017).
Findings obtained in this study show that the first-year students participating in the study have positive procedural knowledge and success levels. Positive knowledge and success levels of these students should be increased, as well. Students' intermediate procedural positive knowledge and success levels can be improved by providing support for students in specific areas. Due to the dynamic relationships of procedural knowledge and the positive effects of question-based activities in understanding the concepts (Zoupidis et al., 2016) or the positive effects of question-based teaching in the development of learning objectives in science education (Vorholzer et al., 2020), these activities can be used to improve the levels of procedural positive knowledge and success. Since creating more than one solution for problems increases students' sense of competence and can be indirectly affected by their procedural knowledge (Achmetli et al., 2019), activities in which more than one solutions of problems can be developed in order to increase their positive knowledge and success levels.
QMT1 is formed from questions in which students' knowledge and success levels can be determined in scientific knowledge and technology applications. In addition, since it can bridge the questions and problems with science and applications, it shows the potential of students to establish a relation between science and technology in the findings obtained from the PST method. In the basic measurement tool, the positive knowledge levels of the students in all the stages of the PST being on a medium level, including the medium level of success indicates that their potential to establish a relation between science and technology is on a medium level, as well.