ABSTRACT
This study aims to examine the link between inequality and diffusion of Information Communication and Technology (ICT). It focuses on a set of 32 African countries. ICT diffusion rate for all the countries was calculated. Two estimation techniques were used: Fractional Regression Model method and Ordinary Least Squares. The results indicate that inequality contributed to the development of ICT in all the countries considered. These results also showed that human capital and the level of development have a positive effect on ICT diffusion. It was also found that institutions play a catalytic role in the adoption and diffusion of ICT.
Key words: Inequality, information technology and communication (ICT) diffusion, Africa, fractional regression model.
Over the last decades, the use of Information Technology and Communication (ICT) has changed significantly, thus giving ICT a prominent place in economic analysis as research fields and growth factor. However, it should be noted that the adoption of ICT is more pronounced in developed countries. According to the Internet World Stats (2017), there are only 353 million internet users in Africa out of a total of 3 billion in the world and the internet penetration rate in Africa is below 30%. This still remains low given the rate of 77% in Europe.
According to the 2012 report of the International Union of Technologies (ITU), subscriptions to broadband often offer an internet connection rate of less than 2 Mbit/s, indicating the poor quality of the connection in these countries. The composite index of ICT Development (IDI) taking into account 11 indicators is two times higher in developed countries. The ranking of 155 countries of the world made on the basis of this index ranks African countries ranking tail overwhelmingly in 2012. This fact is confirmed by the price index of ICT which shows that if the price of ICT services decline in all regions of the world between 2008 and 2011, there are still huge disparities in terms of affordability of ICT.
Prices remain particularly high in Africa, where the value of the price index of ICT was 31.4 in 2011 against 8.8 in the Asia Pacific region, 8.0 in the Arab States and 5.5 in the Americas. Commonwealth of Independent States (CIS) and Europe, where the values ​​of the price index of ICT descended respectively to 2.9 and 1.5 in 2011, are part of the world where ICT services are most accessible (UIT Report, 2012). Given these statistics, it is obvious that the issue of diffusion and adoption of ICTs in Africa is of scientific interest.
These figures led to the observation that there is an uneven ICT diffusion between developed and less developed. A question that emerges from this observation is the unequal distribution due to inequality in developing countries? Referring to the Kuznets hypothesis, inequalities tend to disappear gradually as the benefits of industrialization will be widely distributed throughout the economy. This hypothesis does not appear to be empirically confirmed from the results obtained in developing countries. Indeed, much of the literature studying the relationship between inequality and ICT diffusion concludes that the diffusion of ICTs increases inequalities.
The idea is that the adoption of ICT requires a minimum prerequisite such as a certain level of education, a certain income, and quality of infrastructure. These conditions are generally far from being met by the majority of people of African countries. One might therefore wonder whether it is inequality in these countries that hinders the diffusion of ICT which is a worsening inequality factor (Aghion and Howitt, 1998). For Galbraith and Conceição (2000), the spread of technology does not necessarily produce the expected negative effect on inequality. This vision of the relationship between technological change and inequality is due to a simplified representation of technological change that does not account for the complexity of the relationship between technology and economic performance.
The interest of the present study lies in the difference in the approach used; most of the work seek to understand the impact of ICTs on inequality, but here, we are trying to do the opposite reasoning. Does inequality hinder the diffusion of ICTs in Africa? The objective of the study is to assess the effect of inequality on ICTs diffusion. The assumptions that if the theoretical hypothesis stipulates that ICTs can increase the inequalities and the inequalities can also affect the ICTs diffusion were assessed. The aim of the research is to analyze the effect of inequality on ICT diffusion. It argues that the digital divide is a factor that accelerates inequality. The study has two empirical contributions. Firstly, contrary to the usual hypothesis, the effect of inequality on ICT diffusion is identified and secondly the empirical evidence is about 32 African countries’ sample.
What drives technological evolution?
Many authors assume that technological evolution is skills-based on the idea that there is a complementarity of skills and new technologies. Authors like Krusell et al. (1997), Vindigni (2002) and Acemoglu and Pieshcke (2000) have built formal models along the line of this hypothesis. Employees who know how to use computers at work are better paid than their colleagues, because this computer use requires a higher level of education (Krueger, 1973).
Rosenberg (1972) has argued that the level of employee competence and the status of capital goods sector are two important determinants of the spread of technology in individual companies, as workers and capital goods equipment are critical to the successful adoption and operation of a new invention. If a successful implementation of a technology requires new skills and time, or if it is expensive to acquire the required level of competence, adoption may be slow. Therefore, the overall level of skills available to the company and how skills are acquired are important determinants of diffusion.
From data on US household, Kennickell and Kwast (1997) highlight the role of education, consumer skills and learning in their study of consumer adoption of electronic banking. 70% of all US households used a form of electronic bank in 1995, but only a small fraction of households used the latest, advanced forms of electronic banking such as paying bills. The most common use of electronic banking was to make direct deposits, which is a relatively mature technology, ancient and widely used around the world. This indirectly confirms the existence of a learning effect. As technology develops and improves, more people become familiar with it and they become more comfortable in its use. This accelerates its rate of adoption.
According to Cette et al. (2015), (i) after a long period of sustained growth, ICT diffusion, as measured by the share of ICT capital stock to gross domestic product (GDP) expressed in current prices, has stabilized since 2000 in all four areas; (ii) this stabilization happened at different levels, significantly higher in the United States than elsewhere; and (iii) in all four jurisdictions, the contribution of ICT to labor productivity growth rose significantly in 1994 to 2004 compared to 1974 to 1994. Since 2004, the contribution of ICT to labor productivity growth has fallen off considerably. It only remains positive as a result of the continued advances in ICT performance as proxied by the continued fall in ICT prices.
Ono (2005)shows that GDP per capita and education levels are important indicators, but there is still a significant variation even after controlling them and other economic indicators. In particular, several studies have
shown that the high level of internet penetration in South Korea is considerably higher than expected given their income level, suggesting that South Korea is an exceptional case. At the same time, these studies have shown that internet penetration in Japan is lower than expected for their income level, and that Singapore is performing close to predicted levels.
Technological evolution, productivity and inequality
Acemoglu (2002), comparing changes in the wage structure in the USA, the United Kingdoms and on the European continent, found that wage inequality increased because the labor demand for increasingly skilled increased relatively to its offer. But if this process is audited in the USA and the United Kingdoms, it does not happen the same way in Europe. The author found three possible explanations for this difference. The most important is that technological progress in Europe is not based on skills, suggesting that European countries develop their own technologies.
Following the approach developed by authors like Borghans and ter Weel (2002), the maximum level of wage inequality depends on the distribution of workers across different productivity groups. In an initial phase, wage inequality is increasing and in the long run, these inequalities diminish as productivity gains from computer use. Various factors influence the diffusion of technology (Hargittai, 1999). For Conceição et al. (2005), in an empirical study, inequality has a positive effect on technology diffusion. Bustos (2011) shows that upgrading the skills in business led to an increase in skilled labor demand in Argentina.
Conceição et al. (2005)predict a negative effect of inequality on the new technologies diffusion rate even though the effect of inequality on technology diffusion is positive. The negative effect is explained by the fact that inequality hinders the diffusion of skill-based technological change. So inequality limits the ability of people to adopt and acquire the consumption of new technologies.
Finally, the literature shows that factors like GDP, inequality level, education level determine the ICT diffusion speed, on one hand, and on the other hand, the effect of inequality on ICT diffusion is not exactly identified.
Hypothesis
In this study, the hypothesis that inequalities hinder the diffusion of technology was proposed. Indeed, Conceição et al. (2005) and Beilock and Dimitrova (2003) show that the level of GDP per head plays a key role in the dissemination of technology such as the internet. A fairly large income inequality was expected to hinder the diffusion of technology, because the adoption of ICT requires significant financial resources. If we are in a context where there is great inequality, a significant portion of the population would be without great means and thus not be able to quickly adopt new technologies, which will logically slow the diffusion of ICT.
To test our hypothesis, we limited ourselves to the distribution of both technologies, as internet and mobile phone. In practice, we need to measure the diffusion and capture a variable of inequality. These details are discussed in the subsection on the definition and measurement of variables.
This study focuses on a group of 32 African countries even South Africa included. Since sub-Saharan Africa is a part of the world where issues of inequality and generally development issues are still relevant, we decided to conduct our study on this area, taking also into account data availability.
Definition, measurement and source variables
In this subsection, the details on all of the variables used in this study are shown in Table 1.
Measurement of diffusion variables and inequality
Technology diffusion speed
The spread of technology is not a phenomenon whose shape is uniform in time. Indeed, at first, the technology is new, it is expensive, so that its diffusion rate is slow at first and then after, we enter a proliferative phase where such technology becomes more accessible. This dynamic two-time broadcast can be summarized by an S curve that highlights the fact that technology diffusion is in a non-linear process.
The first indicators that we have to appreciate the diffusion of ICTs are for example the number of subscribers by technology for 1000 people. While it is true that such an indicator has the advantage of giving an overview of the number of individuals who have adopted the technology, this limit will be possible to assess the pace with which, users adopt the technology in question.
To overcome this difficulty, then we must find an indicator that allows one to capture that rhythm. In this logic, so we will calculate the diffusion rates for both technologies object of our study. The technology diffusion rates were calculated following an approach inspired from that adopted by Griliches (1957) and McKnight (2001). Indeed, these authors used a logistic model to study technological innovation. This same approach was adopted by Abdelhafidh and Basma (2009) who studied inequalities and diffusion of ICT.
The logistic model being followed is written as follows:
where Yt is the number of technology subscribers per 1000 people and Ymax is the technological saturation. By remaining in the logic of Conceicao et al. (2005) and Abdelhafidh and Teffah i(2009), we set the technological saturation threshold to be 100% Ymax. b is the coefficient ≥ 0, r is the technological diffusion rate between 0 and 1.
The mathematical form of Equation 1 does not allow one to directly estimate this equation because the variable of interest in this equation is the speed of technological diffusion (r). Therefore, this equation will be turned to bring in a form that allows to estimate the desired speed.
where Zt is the level of ICT speed diffusion, b0 is a constant coefficient, X is a matrix of explanatory variables and εt is the error term.
IDI: Composite index of ICT development
This index is a measure of development calculated by the International Telecommunication Union (ITU) on the basis of international standards defined by experts. This index includes three sub-indices that are the penetration index of the implementation of ICT, the index of the level of effective use of ICT and finally the index of the capacity or competence of ICT.
Gini index
The Gini coefficient is a statistical measure of the dispersion of a distribution in a given population. The Gini coefficient is a number from 0 to 1, where 0 means perfect equality and 1 to perfect inequality. This coefficient is used to measure income inequality in a country. Another measure of inequality is the Theil index. This indicator has the advantage of integrating the contributions of inter and intragroup inequalities in income inequality. This is the main advantage of this index compared with the Gini index. In this article, the Gini index was adopted as a measure of inequality because of the availability of data. This approach is the same used by Abdelhafidh and Teffahi (2009).
ESTIMATION AND DISCUSSION
Two estimation techniques were used in this article. These include Ordinary Least Squares (OLS) and the Fractional Regression Model method. The data used were instantaneous cut. The use of OLS regressions is justified with having as dependent variable the composite index of ICT development. Contrary to the use of OLS for regressions as variables used to explain the diffusion rates is inappropriate. Indeed, several studies in the empirical literature have used OLS to estimate broadcasts speeds. This approach is not the most suitable in that the speeds are variable taking values ​​between 0 and 1. From an econometric point of view, the regression technique suitable for this kind of model is the technique of fractional regression Model.
Regression with human capital variables
Table 2 shows a set of four estimations including three estimates made on human capital variables. The latest estimate is made with inequality income variables. The idea of ​​these regressions is to see first, effect of the capital on ICT diffusion and secondly, effect of inequality on the rate of diffusion of ICT. From the results, it appears that human capital measured here by the primary and secondary school enrollment has a positive and significant effect on the adoption of ICT. Indeed, the composite index of ICT development is a fairly general measure for assessing a comprehensive view of accessibility technologies. It is clear from our results that the more educated the population is, the more developed ICT becomes. This seems logical insofar as more people are educated, that is if they have the intellectual means to appropriate technologies which generally require a minimum level of knowledge.
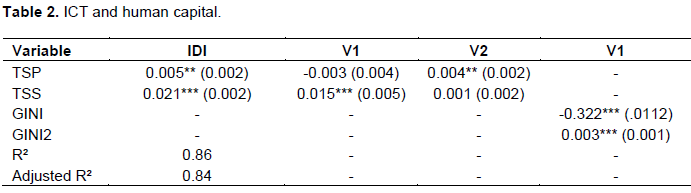
Regarding variable V1 that measures the rate of diffusion of the internet, it should be noted that it is rather the enrollment rate that positively and significantly influences the spread of the internet. This can be explained by the fact that the internet is a technology more or less sophisticated; we need high level of knowledge to better appropriate it.
Regarding the V2 variable that captures the speed of diffusion of the mobile phone, it is clear that it is the primary school enrollment rate that mostly influences its diffusion. This is explained by the fact that increasingly in developing countries, the mobile phone has evolved from a luxury good status to the status of ordinary and necessary goods. In addition, it is evident that we did not need a great education to adopt this technology. This is certainly a minimum of education that can enhance the use of mobile phone.
The last regression of Table 2 relates to the effects of inequality on the ICT diffusion. The measure of inequality used here is the Gini index which is a measure of income inequality. The objective of this approach is to test the hypothesis of Kuznets (1963) on the relationship between inequality and development. Indeed, if we put this hypothesis in the context of ICT development in the context of their distribution, then one might think that inequalities evolve with the development of ICT. This will continue until there will be a reversal of the trend. This hypothesis postulates that inequalities do not have a linear effect and uniform ICT. To test this hypothesis, a specification that integrates the Gini index and its square were adopted. The results of the regression show that income inequality actually has a nonlinear effect on the rate of diffusion of the internet. This effect is not detected significantly on other variables such as mobile phone diffusion rate and the composite index of ICT development. This can certainly be explained by the fact that between the two technologies (cell phones and internet), the internet is the most expensive. Making sure that the effect of inequality is more significant on the distribution of internet.
Regression with the variables of development and complete regression
Tables 3 and 4 present a set of seven regressions. The goal here is to determine the effect of the development level of ICT diffusion in the first place (Table 3) and make regressions taking into account all types of variables in a second time (Table 4).
In Table 3, the first estimate shows that the SRV variable (value added services) has a positive and significant impact on
the IDI. The increase in value-added services in the economy shows a development of the tertiary sector in the economy. This suggests that services are gaining importance in the economy and new technologies are a means to facilitate service provision. This state of affairs explains this result. The same is done with regard to the effect of the Gini index of IDI. This result suggests that inequality is a factor in accelerating the development of ICT. This result indicates that one is from the perspective of the Kuznets hypothesis. This is certain because the countries involved in this study are still in the phase of the first stages of development.
As might be expected, the level of gross national income has a positive and significant effect on the IDI. This result is intuitively justified because technology adoption requires having a certain level of funding. It is therefore logical that the most per capita income level increases in a country more accessible and ICT will be better developed. The variable pp (ICT price basket) which measures in monetary terms, the cost of access to ICT has a negative and significant effect on the index of ICT development. This is in line with a theory justified by the economic literature results. If ICT is analyzed as a normal good, it is normal that as its price increases, its demand decreases.
A third estimate still on IDI shows that the variable (INST), measuring an overall score of institutional quality has a positive and significant impact on the development of ICT. Institutions can be an important factor in the ICT diffusion in the sense that the more a country is better governed with the institutions that guarantee individual and economic freedoms, the more the economy will grow and there will be a better adoption technology, hence this is positive effect.
Regarding the rate of diffusion of the internet, it can be observed that the SRV variables, RNB and PP have positive and significant effect on the spread of the internet. These results seem fairly obvious in the light of the first analysis; however, the positive effect of the price of ICT on the internet diffusion rate is contrary to what is expected. But upon a closer look, this effect could be explained by the fact that the internet as advanced technology is used by people with some financial comfort. It therefore means that once this technology is adopted, users cannot easily let it go, which may explain its development even when its price rises.
Table 4 presents regressions taking into account all categories of variables. These variables thus cover human capital, countries' development levels and purchasing power and the variables measuring social inequalities. In all, it was noted that the estimation results presented in Table 4 confirm the results obtained and presented.
Following different results from the estimates made in this study, attempt was made to say like Conçiacao (2005), there is a positive relationship between inequality and ICT diffusion. It suggests that inequality is in the countries covered by this study, a factor accelerating the diffusion and adoption of ICT. The effect of other variables on the diffusion of ICT has also been examined. The human capital variables were found to have a positive effect on the spread of ICT. Another particularly important result shows that institutions play a vital role in the development of ICT. This result gives more than ever the institutional question in the heart of the debate on the adoption and diffusion of innovations. In terms of public policy, one needs to pursue the actions that target increase in national education enrollment rate and consolidate the institution in order to use it like factor that promotes ICT diffusion, since the literature has shown that better diffusion of ICT can be transmitted to labor productivity growth and then economic growth.
The author has not declared any conflict of interests.
REFERENCES
Acemoglu D, Pieshcke JS (2000). Certification of training and training outcomes. European Econ. Rev. 44:917-927.
Crossref
|
|
Acemoglu D (2002).Technical Change, Inequality, and the Labour Market. J. Econ. Lit. 40:7-72.
Crossref
|
|
|
Aghion P, Howitt P (1998). Endogenous Growth Theory, Cambridge, MA: MIT Press.
|
|
|
Beilock R, Dimitrova DV (2003). An Exploratory Model of Inter- country Internet Diffusion, Telecomm. Policy P 27.
Crossref
|
|
|
Borghans LA, Ter Weel B (2002). The Diffusion of Computers and the Distribution of Wages, ROA working paper Series number 3.
|
|
|
Bustos P (2011). Trade Liberalization, Exports, and Technology Upgrading: Evidence on the Impact of MERCOSUR on Argentinian Firms. Am. Econ.Rev. 101:304-340.
Crossref
|
|
|
Cette G, Clerc C, Bresson L (2015). Contribution of ICT Diffusion to Labour Productivity Growth : The United States, Canada, the Eurozone, and the United Kingdom, 1970-2013. Int. Prod. Monit. (28):81-88.
|
|
|
Conceição P, Faria P, Ferreira P, Padilla B, Preto MT (2005). Does Inequality Hinder the Diffusion of Technology? Preliminary Explor. pp. 1-21.
|
|
|
Galbraith P, Conceição JK (2000). Technology and inequality: Empirical evidence from a selection of OECD countries. In System Sciences, Proceedings of the 33rd Annual Hawaii International Conference on IEEE P 7.
|
|
|
Griliches Z (1957). Hybrid corn: An exploration in the economics of technological change. Econometrica. J. Econometric Soc. 1:501-522.
Crossref
|
|
|
Hargittai E (1999). Weaving the Western Web: Explaining differences in Internet connectivity among OECD countries. Telecomm. Policy 10:701-718.
Crossref
|
|
|
Kennickell AB, Kwast ML (1997). "Who UsesElectronic Banking? Results From the 1995 Survey of ConsumerFinances." Proceedings from the 33rdAnnual Conference on Bank Structure and Competition, Federal Reserve Bank of Chicago pp. 56-75.
|
|
|
Krueger AB (1993). How computers have changed the wage structure: Evidence from microdata, 1984-1989. Q. J. Econ. 108(1):33-60.
Crossref
|
|
|
Krusell P, Ohanian LE, Ríos-Rull JV, Violante GL (1997). Capital-Skill Complementarity and Inequality: a macroeconomic analysis, Federal Reserve Bank of Minneapollis staff report number 236.
|
|
|
Kuznets S (1963). Quantitative aspects of the economic growth of nations: VIII. Distribution of income by size. Econ. Dev. Cult. Change 11(2, Part 2):1-80.
Crossref
|
|
|
Ono H (2005). Digital inequality in East Asia : evidence from japan, South Korea and Singapore. In Asian Economic Panel meeting. Keio University, Tokyo, Japan: Keio University, Tokyo, Japan 4(3):116-39.
Crossref
|
|
|
Vindigni A (2002). Income distribution and Skilled Biased Technological Change, Princeton, Department of Economics - Industrial Relations Sections working paper number 464.
|
|
|
Wooldridge JM (2010). Econometric analysis of cross section and panel data. MIT Press.
|
|